
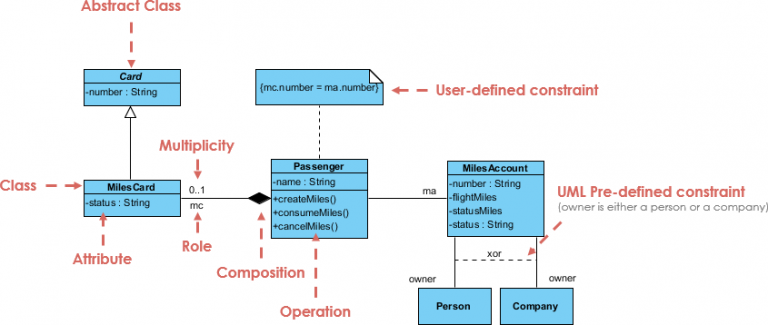
Additionally, asymmetric loss functions and seasonality splitting effectively minimize underestimations despite increasing the overestimation error to some degree. Root-mean-square error (RMSE) results show that removing the anomalies efficiently reduces the underestimation and overestimation errors in all the seasonal datasets. Considering the effect of weather and social factors, seasonality splitting is performed on the three considered datasets from France, Germany, and Hungary containing hourly power consumption, weather, and calendar features. We also apply a density-based spatial clustering of applications with noise (DBSCAN) anomaly detection approach, prior to the load forecasting task, to remove any present oultiers. In this paper, we propose multiple Long Short-Term Memory (LSTM) frameworks with different asymmetric loss functions to impose a higher penalty on underpredictions. However, the power consumption patterns of the residential sector contain fluctuations and anomalies making them challenging to predict. Subjects: Machine Learning (cs.LG) Artificial Intelligence (cs.AI)īuilding an accurate load forecasting model with minimal underpredictions is vital to prevent any undesired power outages due to underproduction of electricity.
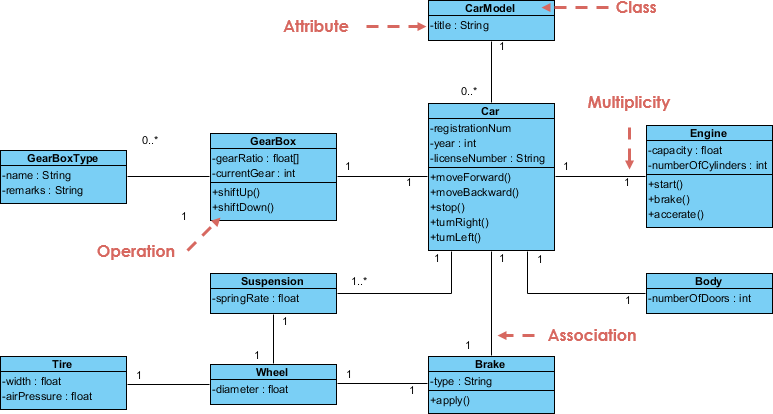
Authors: Authors: Jihan Ghanim, Maha Issa, Mariette Awad.Keyword: detection An Asymmetric Loss with Anomaly Detection LSTM Framework for Power Consumption Prediction
